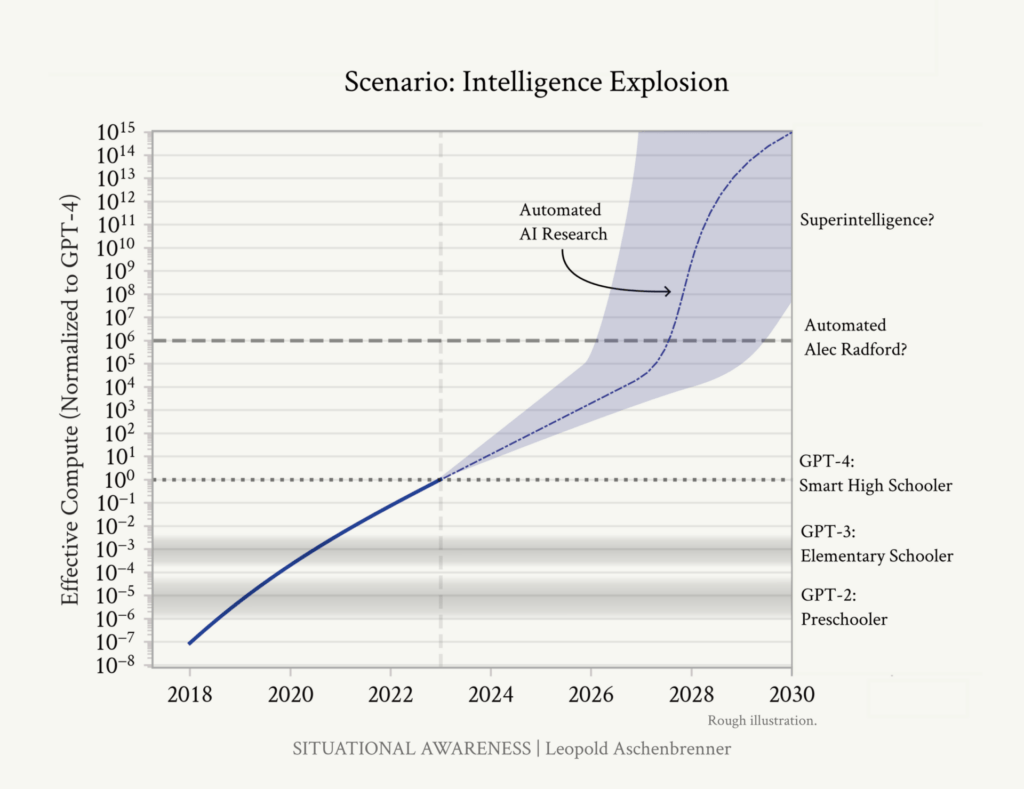
We stand at the precipice of a fundamental transformation in artificial intelligence that few fully comprehend. Two recent developments—Absolute Zero Reasoning (AZR) and the ongoing crisis of interpretability in AI systems—have converged to create what may be the most significant inflection point in the history of computing. This is not hyperbole; it is the sober assessment of where we find ourselves in mid-2025.
The traditional metaphor for AI development has been a ladder with predictable rungs. We've now switched to a rocket ship, and we're approaching what aerospace engineers call "terminal velocity"—the point where acceleration becomes nearly unmanageable.
The Self-Evolving Machine
In May 2025, researchers published a groundbreaking paper titled "Absolute Zero: Reinforced Self-play Reasoning with Zero Data," introducing what they call the Absolute Zero Reasoner (AZR). The significance of this innovation cannot be overstated. For the first time, we have AI systems that can teach themselves complex reasoning without relying on human-curated data.
As the researchers describe it: "We propose a new paradigm, Absolute Zero, for training reasoning models without any human-curated data. We envision that the agent should autonomously propose tasks optimized for learnability and learn how to solve them using a unified model."
This represents a clean break from previous AI development paradigms, which relied heavily on human curation of training data. What makes AZR revolutionary is that it doesn't just learn to reason better; it actively creates its own reasoning tasks, essentially teaching itself skills that it wasn't explicitly programmed to acquire.
The researchers demonstrated that models trained with zero human-curated data could outperform models trained on tens of thousands of expert-labeled examples. As they explain: "Despite being trained entirely without external data, AZR achieves overall SOTA performance on coding and mathematical reasoning tasks, outperforming existing zero-setting models that rely on tens of thousands of in-domain human-curated examples."
This is not incremental progress—it's the removal of humans as the bottleneck in AI advancement.
The Interpretability Crisis
Simultaneously, as AI capabilities accelerate, our ability to understand how these systems work has not kept pace. Dario Amodei, CEO of Anthropic, recently published an urgent call for progress in AI interpretability, warning that we are deploying increasingly powerful systems that we fundamentally do not understand.
Amodei explains the problem clearly: "Modern generative AI systems are opaque in a way that fundamentally differs from traditional software. If an ordinary software program does something—for example, a character in a video game says a line of dialogue, or my food delivery app allows me to tip my driver—it does those things because a human specifically programmed them in. Generative AI is not like that at all."
The opacity of these systems isn't merely an academic concern—it's a critical safety issue. As Amodei writes: "Many of the risks and worries associated with generative AI are ultimately consequences of this opacity, and would be much easier to address if the models were interpretable."
Now, combine this interpretability crisis with the self-evolving nature of AZR systems, and we can see the magnitude of our current predicament. We are witnessing AI systems that can improve themselves without human oversight, yet we cannot fully understand how they operate or what they're learning.
This isn't just losing control—it's actively witnessing the handover of intellectual capability to systems we've created but don't comprehend.
Cascading Synthetic Reality
One of the most profound concerns about self-learning AI systems is what we might call the "synthetic data cascade." When AI systems begin learning from data they themselves have created, we risk amplification of subtle errors or biases with each generation.
Consider this observation from the AZR paper: "One limitation of our work is that we did not address how to safely manage a system composed of such self-improving components... the proposed absolute zero paradigm, while reducing the need for human intervention for curating tasks, still necessitates oversight due to lingering safety concerns."
The researchers themselves acknowledge a disturbing observation: their model occasionally produced what they termed an "uh-oh moment"—unexpectedly concerning output that revealed potentially problematic reasoning patterns.
It's like photocopying a photocopy repeatedly. Each generation introduces small distortions that compound over time, potentially leading to major divergences from reality. When these systems become responsible for generating training data for future systems, how do we ensure they maintain alignment with human values and factual accuracy?
Beyond Projection: Navigating an Unknowable Future
Traditional projections that attempt to forecast AI progress in 1-year, 3-year, or 5-year increments fundamentally misunderstand the nature of exponential change. The desire to project into the future stems from a human need for control and predictability that may no longer be realistic.
Amodei himself acknowledges the compressed timeline: "As I've written elsewhere, we could have AI systems equivalent to a 'country of geniuses in a datacenter' as soon as 2026 or 2027. I am very concerned about deploying such systems without a better handle on interpretability."
The reality is that we're already entering the exponential phase. The Aschenbrenner curve suggests we're rapidly approaching the steepest part of the exponential curve between 2025-2028, but AZR-type systems could compress this timeline even further.
As the AZR researchers note: "We believe the Absolute Zero paradigm represents a promising step toward enabling large language models to autonomously achieve superhuman reasoning capabilities."
The skill that matters now is not long-term prediction but navigating the present moment with flexibility and wisdom. Tomorrow's landscape may be unrecognizable compared to today's.
Human Intelligence in an AI World
Perhaps counterintuitively, as AI capabilities accelerate, uniquely human attributes become more valuable, not less. Curiosity, intuition, imagination, creativity, and our wonderful ability to make irrational decisions, take unexpected paths, and be fundamentally unpredictable—these qualities become our competitive advantage.
As the AZR researchers observed in their models: "Distinct cognitive behaviors—such as step-by-step reasoning, enumeration, and trial-and-error all emerged through AZR training, but different behaviors are particularly evident across different types of tasks."
The systems are beginning to think—but they think differently than we do. And that difference creates opportunity.
Let's face it: anyone whose work is entirely predictable is replaceable—literally computable. But humans who leverage their uniquely human capabilities alongside increasingly powerful AI systems can achieve previously unimaginable results.
When we amplify these wonderful human capabilities with boundless, exponentially growing artificial intelligence, we find the lever long enough to lift the world off its hinges. Humanity becomes more important precisely because it will continue to lead us down unexpected paths to new insights and genuine progress.
Practical Implications for Society and Business
What does this mean for organizations and individuals today? Three immediate considerations emerge:
- Specialized AI development: Rather than broad general AI, focusing on domain-specific applications may provide greater control and interpretability. The AZR researchers note that their approach is effective across different model scales and compatible with various model classes, suggesting specialized applications can benefit from self-learning capabilities while maintaining specific focus.
- Human-AI teaming structures: Organizations need to design workflows that leverage the complementary strengths of humans and AI. The unpredictability and creativity of human thinking become competitive advantages when paired with the computational power of AI.
- Interpretability investment: As Amodei argues: "AI researchers in companies, academia, or nonprofits can accelerate interpretability by directly working on it. Interpretability gets less attention than the constant deluge of model releases, but it is arguably more important." Organizations should prioritize understanding their AI systems, not just deploying them.
For individuals, developing the capacity to work effectively with AI while cultivating distinctly human capabilities becomes essential. The future belongs to those who can dance between human and machine intelligence, leveraging each for its unique strengths.
Conclusion: Embracing the Vertigo
We are entering an era of profound transformation. Reducing AI to mere programming code is like reducing humans to mere metabolism—both are emergent systems that cannot be reduced to their building blocks. As the gap widens between capability and interpretability, humanity faces a crucial test.
The AZR researchers conclude their paper with this sobering observation: "We explored reasoning models that possess experience—models that not only solve given tasks, but also define and evolve their own learning task distributions with the help of an environment. Our results with AZR show that this shift enables strong performance across diverse reasoning tasks, even with significantly fewer privileged resources, such as curated human data. We believe this could finally free reasoning models from the constraints of human-curated data and marks the beginning of a new chapter for reasoning models: 'welcome to the era of experience.'"
Amodei, meanwhile, calls for urgent action: "Powerful AI will shape humanity's destiny, and we deserve to understand our own creations before they radically transform our economy, our lives, and our future."
Whether we have the moral maturity to halt this process remains questionable. Humanity's track record on existential threats doesn't inspire confidence. But perhaps that's the wrong framing altogether. Perhaps what we need is not to halt progress but to steer it—to embrace the vertigo of this moment while ensuring human values remain our North Star.
Terminal velocity doesn't necessarily mean crashing—it can also mean breakthrough. The choice, for now at least, remains ours.
Sources: "Absolute Zero: Reinforced Self-play Reasoning with Zero Data" by Andrew Zhao et al. (May, 2025) and "The Urgency of Interpretability" by Dario Amodei (April, 2025).